At SIGGRAPH 2023, researchers set to debut inventive computational solutions that easily, efficiently overcome complex challenges in physical world
CHICAGO—The vast field of computer graphics and interactive techniques spans the gamut of industries, from gaming and film to biomedical and manufacturing. The core of SIGGRAPH 2023, the 50th iteration of an annual global — and marquis — conference for the brightest and most inventive minds in the field, is a platform to share and showcase the bold, innovative research and products that are changing — and improving — the way we live, work, and play.
Research expected to debut as part of the conference’s Technical Papers program are “ones to watch” — exciting new technologies, ideas, and algorithms that span all areas of graphics and interactive techniques. Particularly noticeable this year is the emergence of research methods that extend beyond the digital world and address creation of real-life content.
“A lot of research in computer graphics has been about determining the best way to visualize various real-world phenomena using computers, and in doing so, there are often details that are elided to fit such complicated things into the computational framework,” says Jenny Lin, a lead author of one of the featured new research projects that will be showcased at SIGGRAPH 2023. Lin and her collaborators have devised a formal semantics framework for machine knitting programs, applying mathematics to describe anything a knitting machine can make.
“Going from virtual representations to the physical world involves addressing details that we often take for granted when going about our daily lives,” she adds. “There’s a very natural connection between these two directions, and there’s something very gratifying about using the language of computers to understand and improve something as tactile and grounded as knitting.”
This year, computer scientists, artists, developers, and industry experts around the world will convene 6–10 August in Los Angeles for SIGGRAPH 2023. As a preview of the Technical Papers program, here is a sampling of three novel computational methods and their unique approaches to real-world applications.
Equipped to Make It Fit
Additive manufacturing, better known as 3D printing, brings digitally designed products to life. It allows for unprecedented freedom of 3D geometries and it gives manufacturers the ability to produce parts on demand, and locally. With 3D printing, supply chain management can be simplified, and it is easier to transition from engineering iterations to full manufacturing.
3D printing is undergoing a transition from being a prototyping technology to being a manufacturing technology. However, the main roadblock is the overall cost of the manufactured part. 3D printing hardware, materials, and human labor all drive the cost of the technology. The drive for higher cost efficiency requires printing in batches where parts are tightly packed in the 3D printer’s build volume to maximize the number of printed parts per batch. One of the main limitations of this process is the limited utilization of the build volume due to the computational complexity of the packing process.
In a collaboration between MIT and Inkbit, a 3D manufacturer specializing in polymer parts, researchers are addressing the complex problem — and headache — of digitally packing many parts in a single container with multiple constraints. To date, many part models are virtually placed in the printing tray, also referred to as “nesting”, and the printer executes the job printing the whole tray. The problem with this process is that the container isn’t densely packed and there isn’t an efficient method to automate and ensure the 3D printers are printing the maximum volume of parts in a designated container.
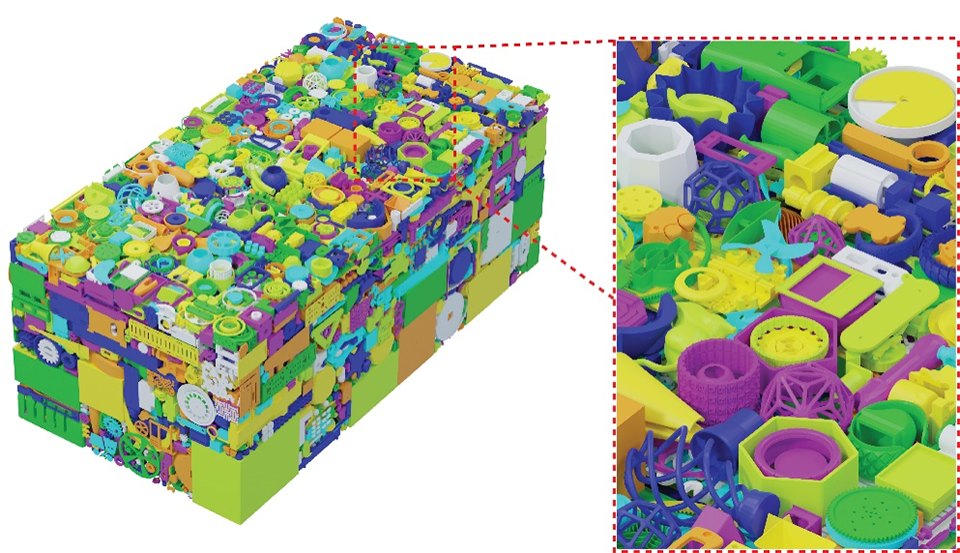
Left: They densely pack the benchmark into a cuboid with a packing density of 35.77%. The packing is free of interlocking. Right: An in-focused view highlighting densely packed objects. “Dense, Interlocking-free and Scalable Spectral Packing of Generic 3D Objects” © 2023 Cui, Rong, Chen, Matusik
The team of researchers, led by Wojciech Matusik, CTO at Inkbit and professor of electrical engineering and of computer science at MIT, developed a novel computational method to maximize the throughput of 3D printers by packing objects as densely as possible and accounting for interlocking-avoidance (between many parts with different shapes and sizes) and scalability. Their approach leverages the Fast Fourier Transform, or FFT, a powerful algorithm that has made it possible to quickly perform complex signal processing operations that were previously impossible or prohibitively expensive.
Coupled with FFT, “our work is making the individual placement of a 3D part into a partially filled build volume as fast as possible,” says Matusik. “Our algorithms are not only extremely fast but they can now achieve print volumes with much higher densities (40% or more). The higher print efficiency will unlock lower cost of parts manufactured.”
The lead author Qiaodong Cui is set to present the new work at SIGGRAPH 2023. The team also includes Victor Rong of MIT, and Desai Chen, research engineer at Inkbit. Visit the team’s page for the full paper and video.
The Refined Knitting Machine
Some may say that knitting is a relatively easy technique, or craft, to learn — and could even serve as a relaxing stress-reducer. Automating the needle-and-yarn technique with machine knitting is well established in the fashion and textiles industries. This has seen a recent surge in popularity due to increased understanding of the scope and complexity of the objects — fabrics, patterns — that can be automatically generated.
While the technique of knitting has been automated, the systems are still struggling with the ability to support everything that a knitting machine can make, as well as generate precisely what a user wants. To date, say the researchers, there is no such system that guarantees correctness on the complete scope of machine knitting programs.
A multi-institutional team of computer scientists from Carnegie Mellon University, MIT, and University of Washington, have created a novel computational framework to optimize machine knitting tasks. Their formal semantics for the low-level Domain Specific Language used for knitting machines provides a sophisticated definition of correctness on the exponentially large space of knitting machine programs.
The researchers applied knot theory to develop their new framework and addressed the key properties humans care about in knitting that are poorly captured by existing concepts from knot theory. To that end, they devised an extension to knot theory called “fenced tangles” as a mathematical basis for defining machine knit object equivalence.
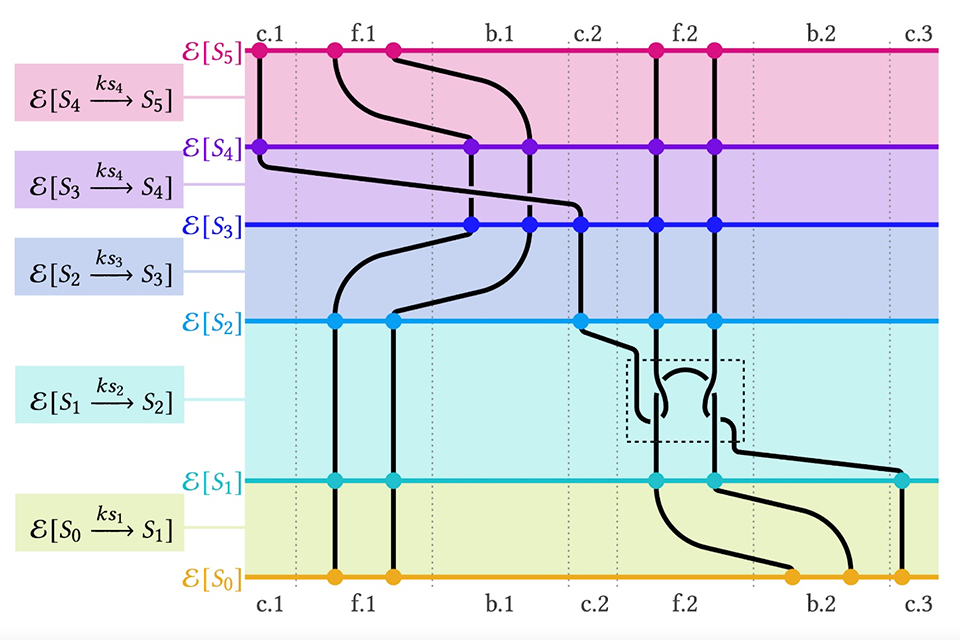
Any knitting machine program denotes a specific fenced tangle, which allows for a formal definition of program equivalence that matches human intuition about knitting. “Semantics and Scheduling for Machine Knitting Compliers” © 2023 Lin, Narayanan, Ikarashi, Ragan-Kelley, Bernstein, McCann
Our method “can describe anything a knitting machine can make: not just your standard sweaters and hats, but also dense, shaped structures useful in architecture, and multi-yarn structures that allow for colorwork and soft actuation,” says Jenny Lin, the paper’s lead author and PhD student at Carnegie Mellon in the lab of James McCann, assistant professor of robotics at Carnegie Mellon and another author of the work.
She adds, “This is important, because as we develop more nuanced systems for generating more complicated knitting machine programs, we can now always answer the question of whether two machine knit objects — the object you want and the object your program makes — are truly the same.”
As a proof of concept, the team has implemented a foundational computational tool for applying program rewrites that preserve knit program meaning. This approach could be expanded for characterizing machine knitting to hand knitting, which is both more flexible and variable as a fabrication technique.
The team behind “fenced tangles” also includes Vidya Narayanan, applied scientist at Amazon who was advised by James McCann at Carnegie, Yuka Ikarashi, PhD candidate at MIT Computer Science & Artificial Intelligence Laboratory, Jonathan Ragan-Kelley of MIT Computer Science & Artificial Intelligence Laboratory, and Gilbert Bernstein, assistant professor of computer science and engineering at University of Washington, and they will present their work at SIGGRAPH 2023. The paper and team page can be found
here .